Dr. Laura Dreessen
VP Conversational AI & Customer Success
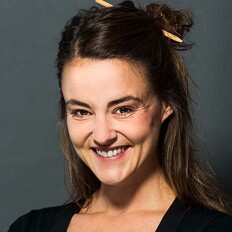
Oana Ciobotea: "How do we use data and AI in VUI.agency’s voice projects?"
Dr. Laura Dreessen: "First and foremost, we use data to train voice recognition since every voice interaction starts with understanding. For this, we need speech data collections to understand how our user’s persona speaks.
When using User Persona design, we create an abstract profile of the user group based on user research. From a linguistic point of view, their language data adds the information on how they speak to an assistant to reach their goals.
In an ideal process, we would provide user scenarios in which users can speak naturally with the assistant to learn how they formulate requests towards an assistant or an AI. The collection of these utterances and audios would let us know about users’ needs and how they individually put them into words so that ASR (Automatic Speech Recognition) and NLU (Natural Language Understanding) can be trained accordingly.
In reality, most voice projects don’t invest the time and money needed for these collections before picking a type of recognition and specifying the intent to be recognized to start a conversation. Usually, we use our expert linguistic knowledge to script training utterances that the assistant is supposed to cover derived from the user persona and the use cases. We need to make sure we train the most probable and natural choices of lexicon, syntax, and register of the respective users in the target language and conversational context.
We select our training data based on the type of recognition. While ML (machine learning) approaches mostly depend on the quantity of data, rule-based approaches would make us choose the most prominent structures to teach the machine.
When planning and implementing custom-built assistants, we have a choice when it comes to the training data, the type of recognition, and even the providers.
In addition to speech data collections and scripting training utterances, our design triggers new utterance data collection in dialogue to optimize and enhance recognition.
One example would be designing open questions and avoiding priming users to respond in specific ways. This leads to new anonymized training utterances that can help improve recognition and enhance the experience.
From my experience working as a senior linguist and VUI architect in the DACH market, I realized that we don’t always need machine learning (ML) and loads of data. However, I know this is a bold claim. Currently, many opinions go against this vision. Because ML is flexible, it can analyze many data and allows for broader conversations with the assistant.
Rule-based systems are more conceptual than machine learning. Language engineers formulate the rules for these systems.
ML automatically does this in a super-fast way, so the experience is more intuitive this way – you can use a broader range of words and sentence structures to express your request. But in some cases, you don’t need a million ways to express your intent to the assistant. For example, when you switch TV channels or the lights, there are not a million ways to do that.
My current vision is to decide what we need in voice recognition and data collection based on the project and the conversational situation.
Secondly, we use different types of data for analytics. Every design is based on a hypothesis of how the interaction between the user persona and the assistant most probably occurs and is perceived as a good experience. To prove such a design hypothesis is right or wrong, we need anonymized data about interactions.
Combining insights such as success rates in recognition and goal fulfillment, among others, gives us the chance to adapt, optimize or personalize interactions. It is only through observing patterns of interaction and user behavior that we can design and implement user-centric conversational applications and meet the needs of people interacting with digital assistants."
"I’m a micro-linguist. Micro-linguists mainly deal with abstractions and instantiations. More precisely, when several instances of something can be generalized under the same abstraction for description purposes. During the ASR and NLU training phase of a digital assistant, we teach machines how individual variants of spoken or written language belong to the same pattern or intent.
Abstracting means that different variants lose individual characteristics to fit the same generalization. The more general the abstraction, the less diversity it covers, the smaller the input quantity, the higher the chance of bias.
Bias in AI happens when algorithms categorize, i.e. derive abstract patterns, from too little and unbalanced data. Hence the necessity of a vast range of diverse data to cover human behavior in all its facets in a non-biased fair way.
When we lack the quantity to capture diversity, we as linguists and designers should provide the quality and balance in training data for machines to avoid wrong abstractions and learning behavior. So when it comes to speech recognition training, make sure you give every user a voice when collecting data. And even more so when scripting it. Ideally, it would help if you had as many socio-linguistic parameters covered in the training data set as possible: age group, gender, origin, register, dialect, conversational situation, or pitch.
The same principle should apply to the audio data used to train an ASR, utterances that serve as a basis for intent design, recognition, and NLU, as well as to interaction patterns you base your design on.
On the level of voice interaction design, we should always be aware that voice designers come up with abstract concepts of conversations between a conceptual user persona.
They are trying to represent as many speaking individuals as possible, and an abstract assistant persona, representing a brand and its values.
To avoid the biased view and make human-machine interaction intuitive and reflecting human conversations, I like to remind myself that my design can never cover all the individual needs of millions of users or come close to the beauty of a human conversation.
When designing conversational assistants, my vision is to reduce the number of individuals represented by the user persona. If you design for a small, dedicated group of users, the risk of introducing bias is lower since you’ll be able to focus on a much lower number of individual needs and characteristics. At the same time, I will need fewer data to cover the machine’s training and less effort to keep a balance in data.
I imagine that this might avoid bias and fulfill the goal of building efficient, context-based, and personal(ized) digital assistance."
"In my opinion, we’re dealing with a huge dilemma. Every data collection or observation during the current age of AI should be based on the persistent effort to avoid data greediness, i.e. collect only as much data as needed in terms of data security, data governance, and data privacy.
At the same time, it should allow for diversity, i.e. provide an enormously huge amount of data to cover varieties or decide on the right balance in data to derive generalized patterns to avoid bias. It’s always up to us how machines learn, interpreting, and generalizing human behavior.
To deal with this dilemma, in a first step, I’d like the opportunity to introduce project managers to linguistic basics, the theory behind the data selection. We should have workshops at the beginning of projects because let’s face it, most people are not linguists, and they don’t need to be.
I want to educate our clients about the technology, how we use the data, and how it influences the behavior of AI. Therefore, my linguist expert colleagues, and I came up with a ‘Linguistics-Based Conversational Design’ course. It explains the most crucial points when defining the conversational partners and the actual conversation or multimodal interaction in a voice project. The course is also aimed at decision-makers and project managers to understand the need for responsible expert decisions.
Businesses are still not so keen on spending big budgets on data accuracy by collecting data to train the recognition before starting a voice project. Training data is often scripted based on our expert knowledge and enhanced step by step by the interaction with the assistant. It speaks to you, and it learns. In this case, our conversation design aims at the correct input, i.e. accurate and non-biased data sets, to train an accurate and secure system. Furthermore, the assistant’s personality sets the scene for responsible voice interaction, which relies on more than just a brand persona or a branded voice.
From a design point of view, we know how to keep data safe and secure. We know how to handle voice and user data ethically correct and find suitable methods to keep our clients’ business goals while delivering an excellent user experience."
It all starts with a good vision and a charismatic character behind the assistant.
"From my personal and expert perspective, we need to reach a point where AI-controlled by voice is a functional tool, not something to replace humans. As such, AI needs to be purpose-driven and goal-oriented. In terms of user-centric voice applications, this means that we should aim to design a good character for every voice assistant that is socially aware and responsible and hopefully also represents the brands in this respect.
At VUI.agency, we try to consult brands to create socially accountable assistants. Visions for voice interaction should combine business goals and use cases derived from our actual daily lives. And not just designing one AI after another outside of the user’s real needs.
Suppose more companies choose a more qualitative approach of use cases and make it available to everyone on the way. Why not create an AI that is just about helping and supporting people with particular needs. We’re not quite there yet, but we are making steps in that direction."
”My vision is to use technology and digitalization to improve the conditions for nature and humanity again. And we need to challenge our AI systems and understand them first to get the best out of them. This is in our hands. Moreover, I want to add that it is vital that clients share this vision or tend towards this vision. The client always needs a vision for their voice projects and digital assistants to control how they behave with data and interact with users.
We help clients understand their brand’s vision and design for it. We also invest in the best possible partners and tools for that to get the best results.
I’m looking forward to the coming times when we will use technology and digital assistants for well-defined nature-oriented goals. There are some approaches like that, not only in voice technology but for example in cryptocurrency with the initiative of including patterns.”